3 Reasons Why Your Company Needs a Recommendation Engine
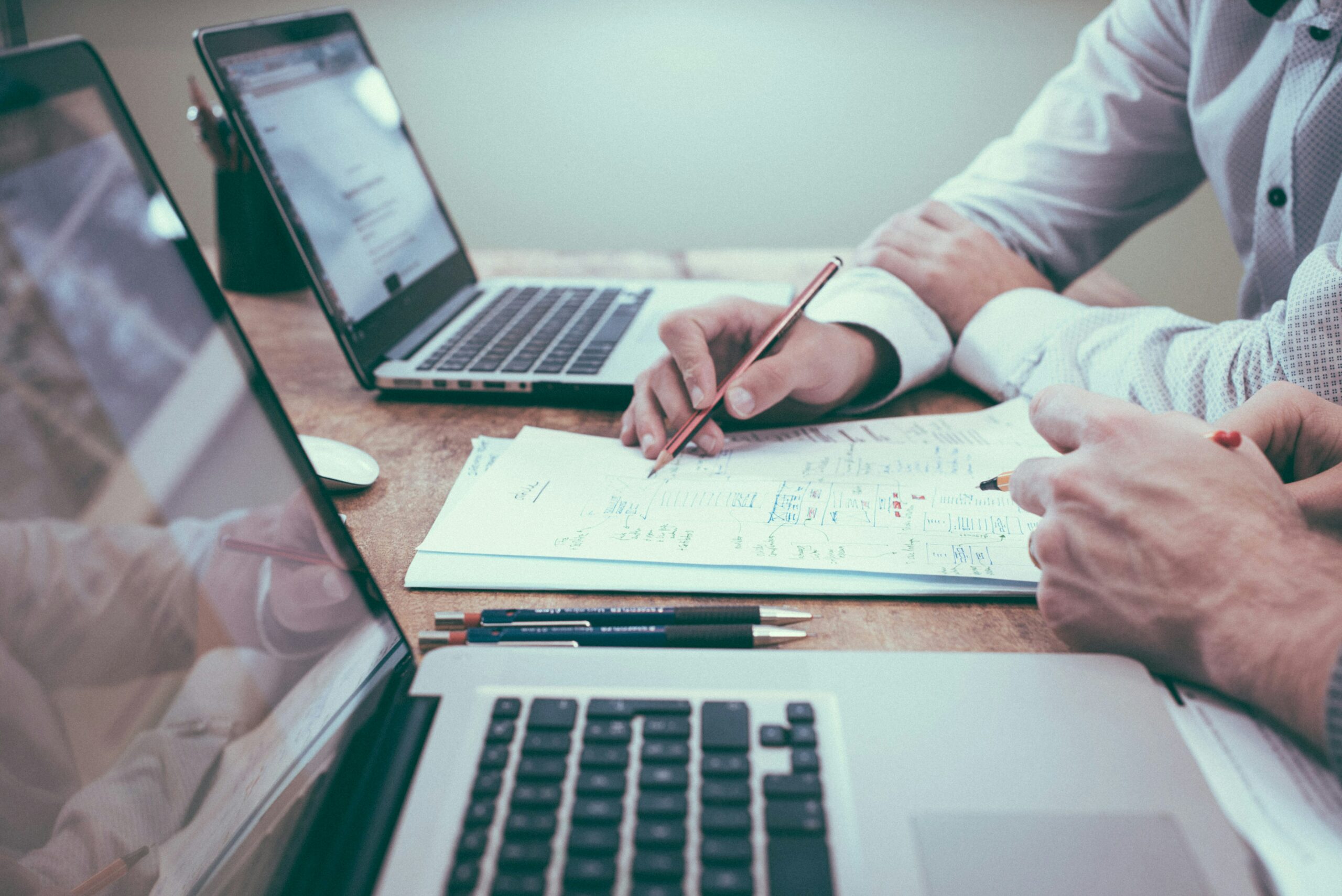
We are willing victims to the power of recommendation engines, but harnessing one is not an instant download, yet. Here’s why you need one and how to get it.
Without fail, we can’t talk about recommendation engines without acknowledging the brilliance of Youtube and Netflix– their recommendation engines are a renowned technique to keep customers engaged and subscribing month after month. Now mimics everywhere make the hot claim to associative-fame saying, “like Youtube for {insert industry x}.” They started a revolution, but despite the number of years we’ve all been willing victims to their power, the advanced algorithms are not quite an instant download that you can apply to your own company yet.
In this article, we’ll lay out some interesting ways recommendation engines are being used and how you can get your hands around the reigns of your own. Beyond the widely used applications of recommendation engines to convert more sales and improve customer retention, we’ll show you even more ways you can make money. We’ve deduced the benefits and recommend these 3 reasons why your company needs a recommendation engine now.
1. Highly personalized product recommendations
This is the best-known use case for product recommendations. If you have a large number of products or an e-commerce website, make product recommendations your focus. You’ve seen it on Amazon, Netflix, and other consumer websites. In B2B sales, a product recommendation engine is also useful because it can provide upsell suggestions for your sales representatives.
2. Optimized Customer Loyalty Programs
According to the 80/20 principle, a minority of your customers will account for a large amount of your sales. These highly engaged customers deserve special treatment, so they keep buying and referring more customers to you. Use a recommendation engine to identify your highly engaged customers and give them special offers and rewards. For instance, instead of offering every customer a 10% off coupon for Black Friday, offer something personalized (e.g., “get a 2 for one offer on all the products you’ve purchased in the past six months”) to your loyal customers.
3. Tailored advertising
In digital advertising, you have the chance to test many different advertising messages. When you have hundreds or thousands of online ads running, identifying winners becomes difficult. That’s where a recommendation engine can help you. For example, Google Ads includes a recommendation engine that provides suggestions on ways to get more traffic by increasing bids.
Case Studies:
On-screen Retail Product Detection and Recommendations
AiBUY is an onscreen shopping tool that utilizes computer vision to recognize products within images and videos, in real-time. Currently, they are focusing on the onscreen detection of fashion items and embedding the shopping tool to enable immediate purchase without being redirected or leaving the screen. A user would simply be watching their favorite content when the AiBUY shopping tool would prompt the viewer with purchasing the items in view.
During an interview with AiBUY’s CTO, Ryan C. Scott, he explained how it works, saying, “Firstly the focus was on detecting a person and their gender. Secondarily adding various other classes e.g. if a woman, then dresses, blouses, etc,” similar to nested categories on most fashion sites. The detected images are then searched within a given retailer’s integrated catalog and the most relevant products are recommended. Scott explains, “By integrating directly with the retailer’s eCommerce platform, we import and synchronize the product information and ensure data like inventory levels and product variance selections are maintained.” Noting one of the latest improvements was “the use of the Annoy library(by Spotify) to improve the performance functionality in searches of nearest neighbors for product classification.” Nonetheless, as Scott humbly remarks “we are always reviewing our codebase to find potential bottlenecks so we can continuously evolve and adapt to the ever-changing outer world.”
Scott continues with more exclusive behind the scenes on how they built it, saying, “The AiBUY system is based on a TensorFlow framework and uses Python as the main language. The training dataset holds about 400,000 images with excellent categorical markup, aggregated and prepared with the help of scripts, supplemented by 1,200,000 images of mixed or poor categorical markup quality from external Affiliate Network companies.” From this rigorous model training, their system is able to recognize clothing worn in your favorite shows, but instead of recommending the next show, it’ll show you where you can buy that swimsuit or other recommended options instantly.
Digital Transformation of Government Documents
Govzilla is a leading data processing company using Big Data and AI to make government data accessible, usable, and valuable to top pharma companies, food manufacturers, medical device companies, and service firms from around the globe. Their system ingests regulatory documents, automatically tags the content to make it accessible, and adds recommended content for researchers as they look for side-effects to drugs, track compliance to FDA regulations, or simply want to look for trends in data.
Govzilla partnered with Blue Orange Digital, a top-ranked AI development agency, to accomplish this goal. They started by replacing manual, outsourced data scrapers with advanced automated ingestion jobs to improve accuracy, scalability, and efficiency.
Then evolved the traditional rule-based text extraction system by incorporating leading Natural Language Processing (NLP) and Optical Character Recognition(OCR) tools. Once the system had the ability to ‘read’ and ‘understand’ the documents, next it needed to file them, and yes, they trained it to do that too. They were able to determine the topics, context, industry, +millions of other categorical tags, and automated the classification of each document along with a range of post-data parsing jobs. Parsing ranged from simple string matching to complex NLP applications including topic modeling, keyword extraction, and semantic understanding. Robotic Process Automation(RPA) was then used to route, store, and index data files throughout advanced ETL jobs. This incredible process managed to move and create a file system while indexing data for quick searches and retrieval automatically.
The result was an expertly trained machine learning model that attempts to replicate, accelerate, and improve the regulatory review process. The model’s inputs and outputs were trained, edited, and audited by industry experts, with years of experience, mimicking their own expertise and critical eye. These automated models are able to correctly parse and identify key terms in documents within a few minutes.
Using this technology, Govzilla will be able “to quantify risk signals about their suppliers, identify market opportunities, benchmark against their peers, and to prepare for the latest inspection trends.” They will be the first to create an objective method for keyword analysis within regulatory deficiency documents. The NLP-driven expert tagging system resulted in the ability to accurately evaluate documents in a few minutes that used to take the human experts multiple hours. The re-engineered data pipeline increased the model accuracy improving the classification and recommended content. Now researchers can spend their time more effectively on finding answers, holding industries accountable, and keeping our society safe and healthy.
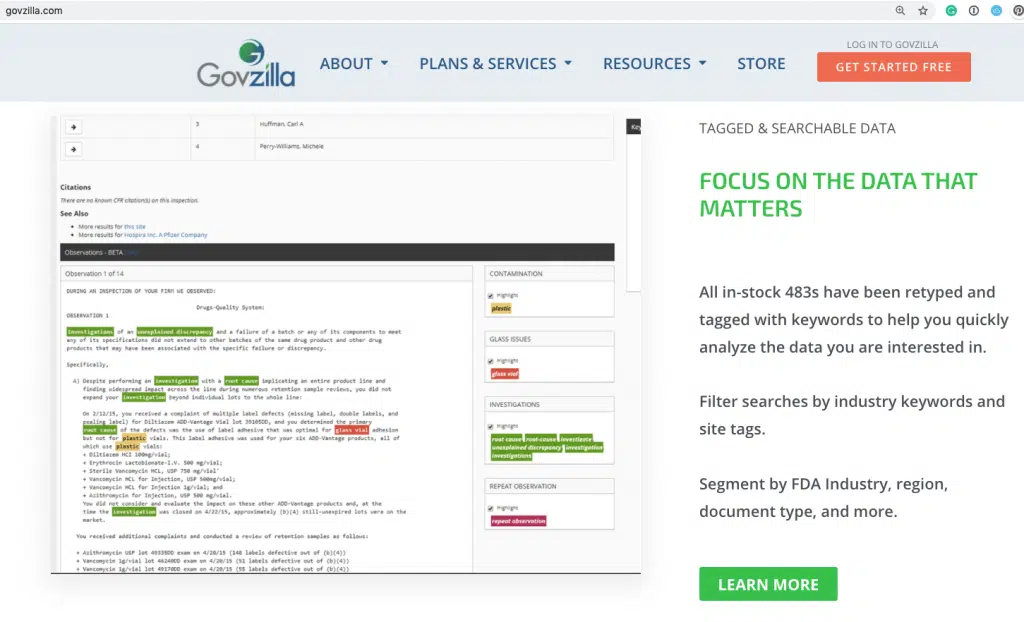
Other Uses Cases:
- Insurance: Recommends associated products/services, like insurance, with recent purchases of new homes and cars.
- Private Equity: Recommends the best investments after ranking all leads.
- Real Estate: Recommends other houses for sale based on search criteria and viewing history.
- Marketing: Recommends personalized offers based on search history(can also suggest the best follow-up method to convert the sale).
- Customer Service: Recommends the correct department for processing reviews, calls, and issues, based on the detected sentiment.
- more advanced: use this model in a chatbot to cut down on expenses.
- Food Delivery: Recommends other restaurants or food items and sends timely offers.
- more advanced: predicts the acceptance rate of offers and balances the delivery demand with customer satisfaction.
- Retail: Recommends products, predicts campaign success and reports supply chain demands.
- Entertainment: Recommends related articles on a popular magazine’s website.
- User-generated content: Recommends categories to organize the topics properly via keyword matching.
- more advanced: automates this process.
- more advanced: beyond keywords, understand phrases, and context with NLP.
- Pharma-Forum Monitoring: Recommends content based on their searches, statements, and questions posed.
- more advanced: Tag critical information to inform drug companies of noticed correlations of side effects.
- Finance: Recommends the best loan provider for a homeowner’s or broker’s location and situation.
- Cooking: Recommends recipes based on ingredients, best reviews, and previous recipe viewing history.
- Automotive: Recommends the best car for a given user. Could also recommend service/parts for described issues through a chatbot.
- E-learning: Recommends courses and content based on the user’s viewing history, level, and goal.
- Business Intelligence: Recommends visuals for given data inputs through Smart Dashboards.
- Health and Wellness: Recommends personalized exercise videos, recipes, and content.
- See more Case Studies
The Fast Way To Bring Recommendation Engines To Your Company
Developing a recommendation engine takes data expertise. Your internal IT team may not have the capacity to build this out. If you want to get the customer retention and efficiency benefits of recommendation engines, you don’t have to wait for IT to become less busy. You can partner with a co-development firm, like Blue Orange Digital, a top-rank AI consulting and development agency, to bring results faster and with less investment.
Article originally published on Datafloq.com