AI in Commercial Real Estate: From Hype to Impact
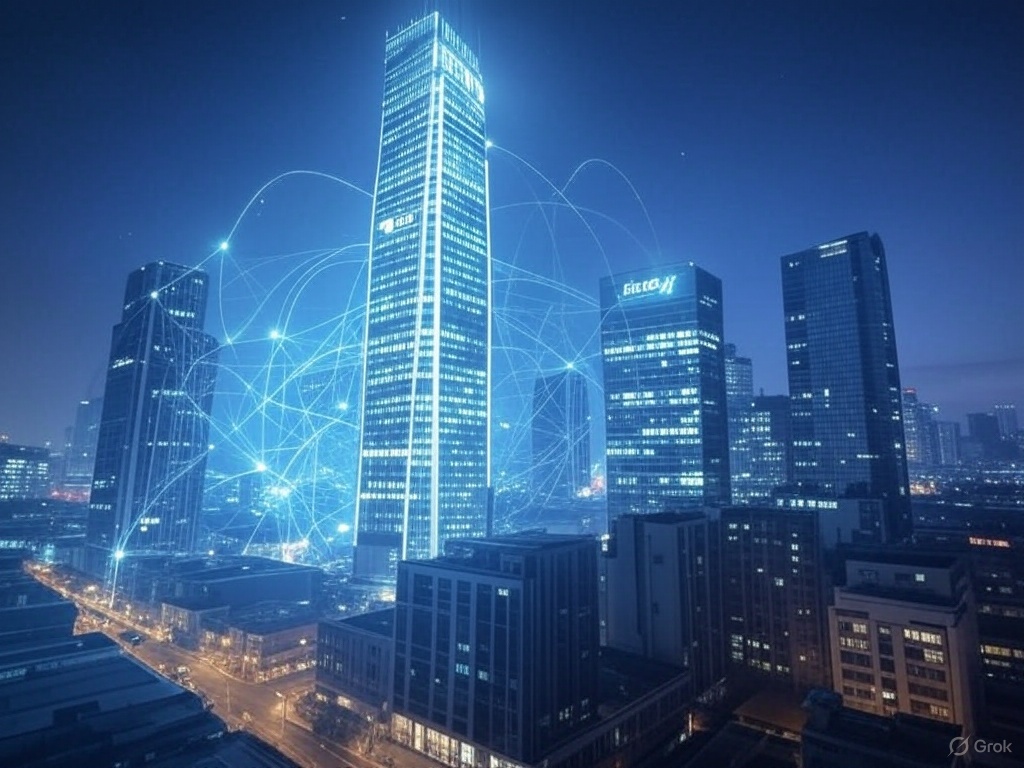
Introduction
The commercial real estate (CRE) industry is abuzz with talk of Artificial Intelligence (AI) and Large Language Models (LLMs). But how far along are we really in leveraging these powerful technologies? A recent webinar, “From Hype to Impact: How AI and LMS are Driving Commercial Value in Corporate Real Estate,” brought together experts to discuss the current state, challenges, and opportunities of AI in CRE. This article summarizes the key takeaways from that discussion, offering insights for CRE professionals looking to navigate the AI landscape.
The Early Innings of AI Adoption
CRE is still in the early stages of AI adoption. While other sectors like finance, healthcare, and retail have made significant strides, CRE is just beginning to explore its potential. There’s a need to move beyond simply layering AI into existing SaaS tools and start thinking about larger, more bespoke initiatives. One reason for this slower adoption is a lack of fundamental understanding of AI within the industry. Too often, the focus is on immediate use cases without grasping the underlying technology. This can lead to “burned fingers syndrome,” where early failures discourage further experimentation.
Defining and Achieving Commercial Value
What does “commercial value” from AI even mean in CRE? A useful distinction can be made between operational and strategic value. Operational value refers to efficiency gains achieved through automation and digitization, such as report generation and data movement. Strategic value, on the other hand, involves transforming business practices for competitive advantage, for example, by identifying high-growth markets. While automating reports is helpful, it won’t fundamentally change your business. CRE needs to define the intended outcome of AI initiatives to truly evaluate their effectiveness.
However, significant value can also come from smaller, incremental AI-powered workflows that address consistency problems and save human hours, as one expert pointed out. It’s also been noted that digitizing foundational processes is a prerequisite for strategic AI applications.
Why the Slower Pace?
Several factors contribute to CRE’s slower tech adoption. Newer employees expect technological solutions, forcing a change in mindset. CRE has traditionally been a relationship-based business, where technology hasn’t always been central. However, the new workforce is demanding data-driven tools. Historically, CRE has prioritized asset acquisition over technology investment. However, increasing investment in real estate tech signals future innovation.
Navigating the Evolving Tech Landscape
The complexities of build versus buy decisions in the rapidly evolving AI landscape are significant. The immense VC funding flowing into AI startups makes it challenging for individual firms to compete in building cutting-edge solutions internally. A mix of built and bought solutions is likely, influenced by factors like time-to-market and available expertise. Managing AI embedded in various systems also adds complexity.
Data Security and Governance
Data security and the use of public LLMs are major concerns. Some firms are implementing internal LLMs to mitigate privacy risks and actively discourage the sharing company data externally. Many are developing AI guidelines (evolving into formal policies) in partnership with HR, emphasizing education and awareness around data privacy. Josh discussed emerging technological design patterns for LLM implementation that involve making models narrower in knowledge and capability and tying them to identity and access management protocols for better data control within an organization.
AI Fundamentals and Data Quality
It has been strongly emphasized that “AI is mathematics. Machine learning is statistics.” Many in CRE lack a fundamental understanding of these concepts. There needs to be a focus on understanding the technology, its capabilities, and its limitations before jumping to use cases. The critical importance of data quality and a robust data infrastructure as foundational for successful AI implementation cannot be overstated. It is not possible to have an effective AI without clean and well-managed data.
Iterative Development and Managing Expectations
The iterative nature of AI development and the need to manage expectations around failure are key. One expert framed iteration as the path to success in software development, including AI. It’s important to train users that AI outputs may not always be accurate and that human expertise remains crucial for review and decision-making. The need to differentiate between AI applications focused on improving operational efficiency and those aimed at achieving strategic advantages is paramount. The development and evaluation processes differ significantly for these two categories.
Conclusion
AI offers tremendous potential for the commercial real estate industry. By focusing on understanding the technology, prioritizing data quality and security, and clearly defining objectives, CRE firms can move beyond the hype and unlock real commercial value. Embracing a strategic and informed approach to AI implementation and recognizing both its potential and its limitations is essential for success.