Boring Machine Learning Everyone Should Get Excited About
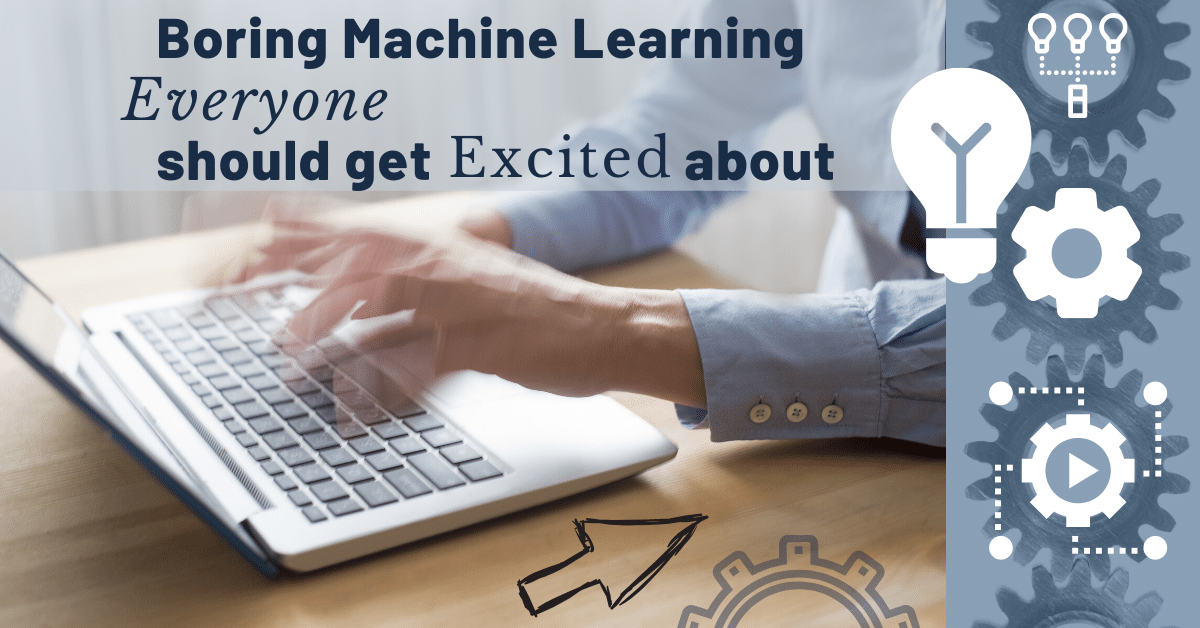
Machine Learning has become a strategic focal point for businesses that wish to find innovative solutions to their most complex problems. Examples of successful applications can be found in every industry and AI solutions are causing ongoing disruption to business models.
But there is a very simple reason why the predictive power of machine learning algorithms is so valuable for businesses: it helps them cut down on costs and resources associated with the most tedious and unrewarding tasks. Let us take a closer look at a few examples of “boring” tasks that can be delegated to machine learning tools.
Automated Document Processing & Analysis
Businesses deal with large amounts of documents and written content (emails/correspondence) originating in their daily operations. Both internal and external channels are sources of information that can be collected, analyzed and turned into valuable business insights.
Document understanding tasks can be simple (e.g. search and match) or complex (topic modeling, keyword extraction, semantic understanding), depending on individual business use cases. However, the unstructured nature of document data makes it challenging to understand and process manually. Such tasks are tedious and overwhelming without an automated analysis and parsing approach. Some may even be unsolvable due to the large data volumes and large processing times involved.
Computer vision methods (such as OCR) and NLP tools are known solutions for various document understanding tasks. They have evolved from traditional rule-based approaches and can be trained on large amounts of data. Automated parsing results in faster document processing times and increased accuracy. Also, they make it straightforward to scale when more processing power is needed.
Intelligent Conversational Interfaces
Businesses in all sectors face a constant need to offer high-quality customer service. Be it assisting online shoppers, providing answers to technical questions, or suggesting products, it is all about serving customers with the information they need when they need it.
Traditional customer and technical support teams need to deal with large volumes of routine inquiries while having limited availability. This usually results in long interaction times, decreased customer engagement, and wasted resources. Also, outdated communication channels may not satisfy customers’ needs for an immediate response, which may negatively impact sales and marketing results.
Intelligent conversational agents can deal with both voice and text inputs and can infer responses to natural language queries. They learn from the customers’ past preferences and can provide personalized support in real-time, with permanent availability. When chatbots deal with the basic queries, they free up time for the expert human operators to tackle more complex issues.
The advantages for businesses are clear: an intelligent conversational solution ensures that customers can be assisted at any time, on any channel. Personalization and interaction are known to improve customer engagement. Also, there are no limits to the volume of queries that can be handled. When more chatbots are needed, more can be deployed.
Data Extraction and Information Retrieval
Apart from leveraging their own data for valuable insights, many businesses also rely on online information for their B2B or B2C processes. Information published via the internet is available in different formats (HTML, XML, PDF, CSV) and can originate in a wide variety of sources. Ecommerce transactions, news articles, RSS feeds, marketing & sales reports or customer feedback forms are only a few examples.
But handling such a variety is impossible by manual processing. Especially when also considering the huge data volumes and its velocity. Manual data extraction is an inherently error-prone and inefficient process. Searching for the data of interest in a source web page, copying and pasting it into a structured format (usually spreadsheets) for further analysis is not only cumbersome but also time-consuming.
Automated extraction frameworks were built to prevent such waste of time and resources. They can handle heterogeneous data sources and multiple formats and can be configured to stay up to date with ever-changing API specifications. The value for businesses is again clear: by removing time-consuming, error-prone, and inefficient processes they enable human staff to focus on more important business aspects. The predictive superpowers of ML tools have changed the way businesses think about, rely on, and implement artificial intelligence solutions. Achieving more with fewer resources is now possible and data assets can be turned into tangible operational improvements.
Blue Orange Digital has vast experience using predictive technology for otherwise boring and wasteful tasks. You can read more about our document processing solution and about our revenue prediction case study.