Letting Your IoT Customer Data Work For You
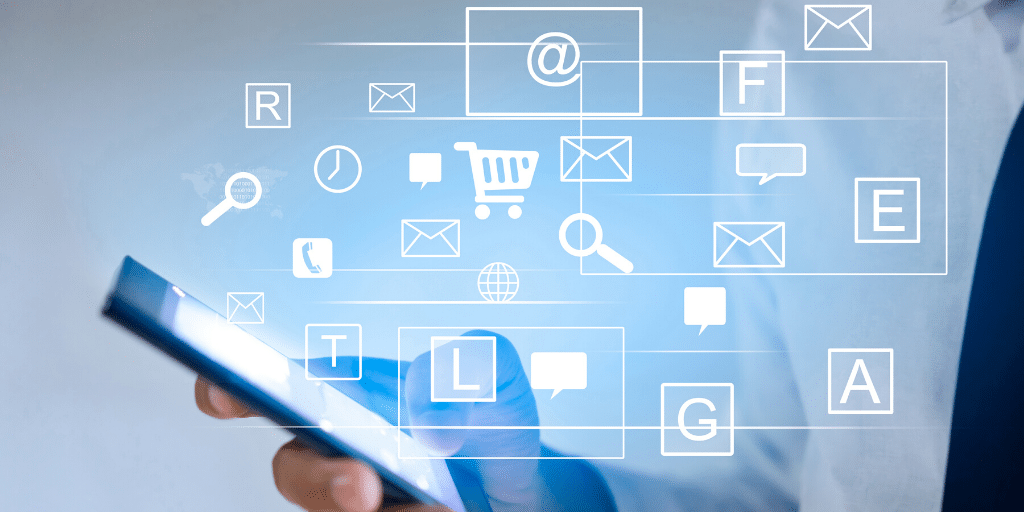
Self-driving cars, payment systems, wearables, cameras, IoT devices, smart industrial machines, and online tools. From software to hardware, intelligent services and connected smart devices played a major role in the data-driven industrial development of the past decade. Nowadays, AI and machine learning analytics allow organizations to discover a new value of such smart devices. Since data in itself is a crucial asset for technically capable organizations, the value of such smart devices is tightly related to the data they generate. And to how you make use of that data.
There are two main directions in which data analytics can be beneficial to an organization. Firstly, internal business workflows can be optimized via the gained data insights. Secondly, customer-oriented product development efforts can be better directed when user data insights are taken into account.
Internal Benefits
Insights gained by means of data analytics offer a chance to optimize both costs and revenue. Labor expenses, machine repair & rent fees and even advertising budgets are only a few examples of operational aspects that may easily be improved if data is put to work.
MGI research reports cost reduction of 5 to 15 percent in the case of manufacturing companies that implemented operational analytics. Regarding revenue, data and analytics allow a business to explore new markets, improve distribution channels and properly target customer segments. Moreover, operational decisions do not need to be made in the dark anymore: data-literate industry leaders are able to translate data insights into increased employee productivity and keep operational costs to a minimum. As such, data analytics is an extremely powerful tool that translates to a non-negligible competitive advantage.
External Benefits
Analyzing customer data creates valuable insights that allow building increasingly personalized products and services.
From the insurance and fintech sectors to health care, retail, and logistics, some of the most useful insights come from behavioral analytics. Data captured in both online and physical environments shed light on the actions of your customers and allow the development of useful features that further improve your products. For example, an online retailer could not implement a functional product recommendation system without fine-grained behavioral data at hand. Products that match the needs of your customers ensure a higher retention rate since they actually build value for the users. Consequently, your product is more likely to be recommended and attract new users. Without thoroughly analyzing and drawing insights from user data, such strong benefits would be impossible to achieve.
But all these benefits are only reserved for those that do the hard work and try to get the customer data to work for them.
How can you let your own customer data do the work for you?
The highest performance of the modern analytics framework is only achieved when integrating multiple data sources, i.e. bringing in orthogonal data.
The power of orthogonal data is in the fact that it complements existing data sources and helps create a more detailed view of the interaction between your product and its user. Below are a few simple steps you can take to start integrating multiple data sources into your analytics solution.
Prevent data waste i.e. perform analytics on already collected data
Let’s take an imaginary example of a plant manager in charge of a production facility. The manufacturing process assumes that humans and robotic arms perform collaborative tasks. The robotic arms are already equipped with sensors informing about current system status and environmental variables, such as humidity, temperature & vibration. The robotic arm is also already tracking and logging the user interactions. Aiming to lower the operating budget, the plant manager sets a specific goal (e.g. lower the number of robotic arms) and decides to hire a data analytics team to help find potential glitches in the workflow. While sending the data to be analyzed, he completely ignores the user interaction data and only asks for insights based on the system usage data.
Such a waste of data!
While humans are unable to discover hidden correlations, the power of machine learning and predictive modeling allows us to achieve just that: unexpected outcomes may be learned by the algorithms when they are fed with seemingly unrelated data.
Aggregate multiple data types, even if they come from different sources
The IoT world is populated by smart devices that not only collect large amounts of sensor and usage data but are also able to communicate with each other. Advanced wireless protocols such as Zigbee provide a secure mesh network for smart devices to exchange data. The processing and analysis of device data can be done either at a device-level (the so-called edge computing) or in the cloud. The flexibility of computing options and affordable analytics solutions has enabled IoT platforms to collect ever-increasing amounts of data. This can, for example, break barriers at the application level:
“Connected light fixtures, which sense the presence of people in a room and have been sold with the promise of reducing energy usage, generate “data exhaust” that property managers can use to optimize physical space planning”
However, heterogeneous data sources pose a modern data analytics challenge: specialized analytics tools are needed to be able to process, aggregate and analyze different data types and formats.
Here at Blue Orange Digital, we employ the data lake model, which allows us to build end-to-end analytics solutions without worrying about heterogeneous data types.
Integrate third-party data
Valuable insights from data analytics may be obtained when integrating third-party data, i.e. data originating from sources not directly linked to your application scenario. Examples of external sources are social media feeds, mobile geolocation data or even video feeds. Such orthogonal data sources may give meaning to previously unusable sensor data and allow running analytics on a large scale. As an example, AGT International’s Wearable Analytics Lab has augmented motion sensor data extracted from smartphones with video feeds of facial expressions and audio streams in order to build realistic social analytics models of crowds. This shows once again that there are endless possibilities when unleashing the power of orthogonal real-time data.
Are you ready to join the industry leaders and employ machine learning and advanced analytical tools for your business needs? We are happy to crunch your data and help you gain crucial insights about your operations and further improve your products.
Drop us a line and let us unleash the power of your customer data!