Bold NLP and OCR: Use Cases
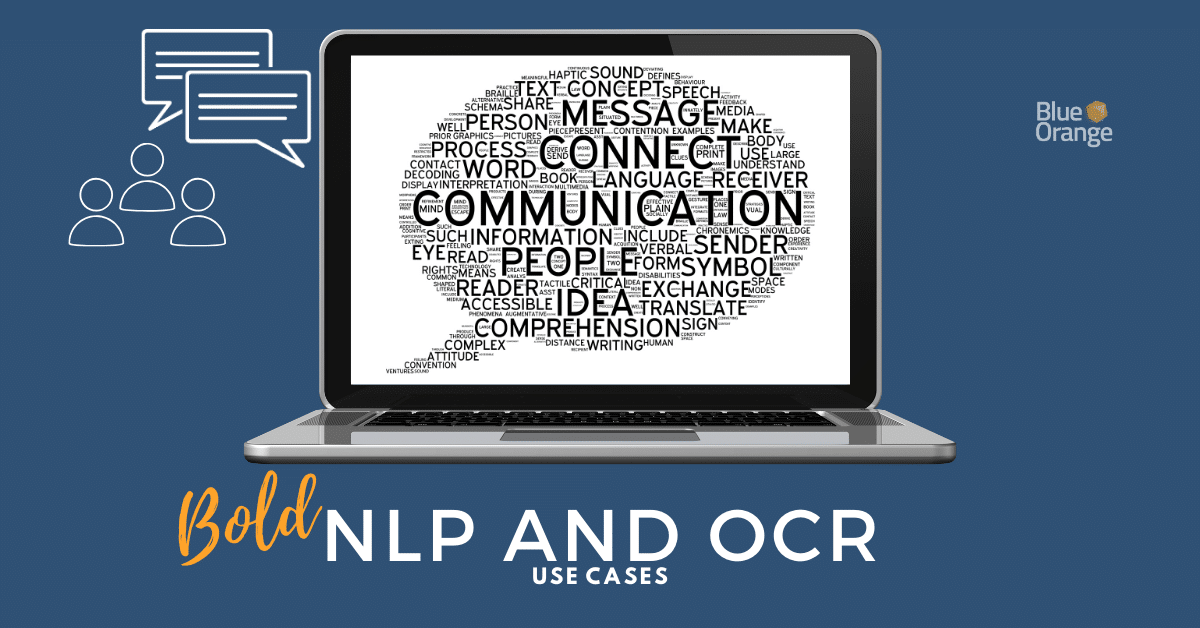
Last time we’ve covered some boring machine learning use cases, showing how algorithms can leverage data and solve tasks in an automated manner. Today we take a closer look at two machine learning disciplines that enable advanced automation: Natural Language Processing and Optical Character Recognition.
Natural Language Processing
NLP is an umbrella term defining all (machine learning) tasks aiming to understand and process human-generated text or speech. Among all machine learning disciplines, NLP faces a particularly challenging aspect: the data originating in human language and communication is highly unstructured. Online reviews, internal communication emails, newsletters and customer service logs are all examples of such unstructured data. When businesses need to handle it on a daily basis, they are not only interested in solving simple tasks (e.g. keyword extraction), but in understanding the actual meaning of the content. NLP algorithms can decipher that meaning and provide valuable interpretations of human language.
NLP-based applications are deployed by businesses in a variety of scenarios. Take the example of the real-estate agency that wishes to evaluate tenants’ sentiments towards property management. NLP models trained on reviews written in natural language can help identify the property quality, ongoing issues, and overall investment potential. A study covering the use of AI and ML in the real-estate sector has also identified another popular NLP-powered solution: 24/7 customer service via chatbots and virtual assistants. Having natural, personalized conversations with humans is made possible by advanced Natural Language Generation models. This gives agencies an opportunity to improve the interaction experience of their potential customers (or tenants). Last but not least, NLP tools are ideal information extraction tools. They can process, analyze and extract meaning from human-generated text and speech content. Such applications are nowadays as ubiquitous as spam filters.
NLP models are extremely powerful due to their capacity of handling highly unstructured data (such as natural language) and turning it into structured, analyzable data. This means that large volumes of text information can become a valuable source of business insights.
Optical Character Recognition
OCR technology has developed with the business need for capturing data from physical documents. Letters, invoices, printed contracts or even images are examples of documents that need to be managed as part of daily business operations. However, high document volumes turn the most basic tasks (such as searching) into extremely time-consuming and costly endeavors. OCR tools create digital copies of said documents and can extract data into structured formats (i.e. databases). This makes the data readily available for further processing and enables quick sorting, searching and editing of the stored information.
OCR applications are encountered across industries. An example coming from the banking sector is the handling and processing of cheques. OCR tools perform automated cheque clearance (i.e. scanning, text conversion, and signature matching) and save time for all parties involved – the bank, the payer, the payee. In the legal sector, an example is the possibility to search across a large number of documents. OCR solutions can handle large volumes of documents and enable fast access to information, right at the moment when it’s needed. Accounts payable is another example that is relevant for companies serving a large number of customers, such as the ones in the energy sector. Scanning invoice contents and storing them as key-value pairs into a database is a well-known approach to make invoice data ready for further electronic processing.
Examples, can, of course, be found across all industry sectors. The bottom point is: OCR technology redefines the way businesses operate with and handle documents. When digitized document information is available in a database, it becomes ready for all kinds of further processing: searching, editing, and even translating.
Business Benefits
Gartner identifies the disruptive potential of NLP technologies since they enable text analysis and speech recognition applications. Powered by NLP, businesses can develop solutions that:
- replace time-consuming manual processing with automated solutions
- transform unstructured data into structured, analyzable data formats
- turn text & speech data into valuable assets
Similarly, OCR tools have become a top priority for businesses looking to streamline their document processing workflows. The use of OCR technology brings the following advantages:
- Saves costs on storage space
- Makes information easily available
- Reduces the costs associated with manual processing
The key takeaway is clear: NLP and OCR make it possible to streamline processes and improve operational efficiency. They assist businesses in their data transformation journey while helping them cut down on operational costs. This turns them into important strategic capabilities for any company that wishes to leverage their data assets.
Can NLP and OCR solutions be developed for your business use case? How to get started with an NLP solution? What processes can be automated via OCR?
Do you have any related questions? From HR to health care, the Blue Orange Digital team has extensive experience with OCR and NLP based solutions.
Get in touch and we are happy to provide you with answers!